The Transformative Power of AI and Big Data in Stock Trading: A 2025 Perspective
In 2025, the landscape of stock trading is vastly different from a decade ago, thanks to two major technological revolutions: Artificial Intelligence (AI) and Big Data. These technologies have not only changed how markets operate but also how investors think, strategize, and act. This comprehensive guide explores the depths of AI and Big Data’s influence on stock trading, from enhancing decision-making processes to predicting market trends with unprecedented accuracy.
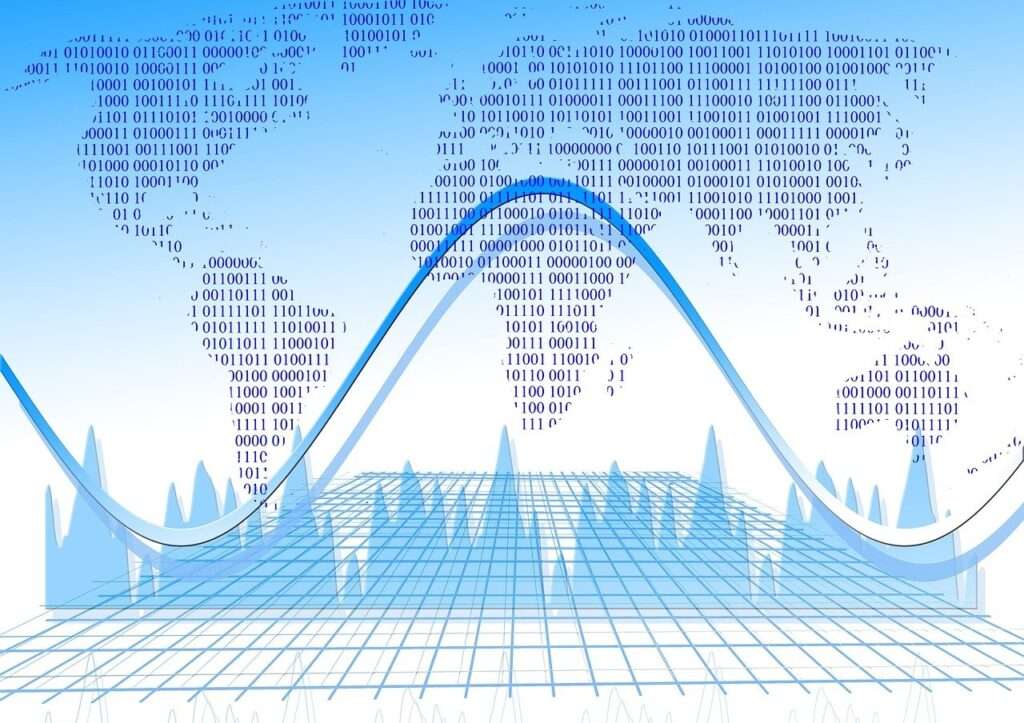
What is AI in Stock Trading?
AI in stock trading encompasses a wide array of technologies:
- Machine Learning: At its core, machine learning involves training models on historical data to predict future market movements. Supervised learning can predict stock prices, while unsupervised learning might identify market segments or anomalies.
- Neural Networks: These mimic the human brain’s structure, allowing for complex pattern recognition in stock data, which is crucial for understanding market dynamics that are not immediately apparent.
- Natural Language Processing (NLP): With NLP, AI systems can interpret news, earnings calls, and social media sentiments, offering insights into how information might affect stock prices.
- Reinforcement Learning: AI learns optimal trading strategies by simulating market scenarios, akin to learning through trial and error, which is particularly useful in dynamic market environments.
Big Data: The Fuel for AI’s Engine
Big Data complements AI by providing the necessary volume, variety, velocity, and veracity of data:
- Volume: Markets generate petabytes of data daily, from price ticks to global economic indicators.
- Variety: Includes structured data like financial statements, semi-structured data like XML feeds, and unstructured data like analyst reports or tweets.
- Velocity: Real-time data streams require AI systems to process information at high speeds for immediate decision-making.
- Veracity: Ensuring data accuracy is paramount since AI’s effectiveness is contingent on the quality of the data it processes.
Key Applications of AI in Stock Trading
- Algorithmic Trading: AI enables the execution of trades at microsecond speeds, capitalizing on minute price changes through high-frequency trading (HFT).
- Predictive Analytics: AI algorithms predict stock movements by analyzing historical data, market news, and even satellite imagery for economic activity.
- Sentiment Analysis: By processing vast amounts of text, AI can gauge market sentiment, which is often a precursor to price movements.
- Risk Management: AI models simulate countless scenarios to assess risk, aiding in portfolio rebalancing or hedging strategies.
- Automated Portfolio Management: Robo-advisors use AI to manage diversified portfolios, adjusting them based on real-time market conditions and user preferences.
Case Studies: AI and Big Data in Action
- Renaissance Technologies’ Medallion Fund: Its secretive, data-driven approach has kept it at the forefront of AI trading, with some of the highest returns in the industry.
- Quantopian: Now part of Robinhood, it once allowed a community of developers to create and test AI trading models, democratizing sophisticated trading strategies.
- BlackRock’s Aladdin: More than just trading, Aladdin uses AI for comprehensive risk management, influencing investment decisions across BlackRock’s vast asset management empire.
- Two Sigma: Known for its heavy reliance on data scientists, Two Sigma uses AI to develop trading strategies based on complex mathematical models.
- Citadel Securities: They leverage AI for market making, executing billions of trades daily with algorithms that adapt to market conditions in real time.
Challenges and Considerations
- Ethical Dilemmas: There’s a growing concern about AI’s potential to manipulate markets or create flash crashes if improperly managed.
- Data Privacy: The use of personal data in trading algorithms raises significant privacy issues, necessitating strong data protection measures.
- Over-Reliance on AI: There’s a risk that over-dependence on AI could lead to neglecting the human element in trading, like intuition or ethical considerations.
- Regulatory Hurdles: AI systems must navigate an ever-evolving regulatory landscape to ensure compliance with trading laws and ethical standards.
The Future of AI in Stock Trading
- Personalization at Scale: AI will tailor investment strategies not just based on financial data but also on behavioral patterns and personal values.
- Quantum Leap: Quantum computing could revolutionize AI by processing complex financial models at speeds currently unimaginable, leading to more accurate predictions.
- AI-Driven Financial Products: Expect more investment products designed around AI’s capabilities, like ETFs that adjust based on AI predictions or AI-managed funds.
- Ethical AI Frameworks: As AI becomes more autonomous, frameworks for ethical decision-making will become crucial to ensure AI aligns with human values.
- Integration with Global Systems: AI will increasingly connect with global economic indicators, IoT data, and even environmental sensors for a holistic market view.
AI Beyond Stock Trading
- Cryptocurrency Markets: AI’s role in analyzing and predicting the highly volatile crypto markets, where traditional financial models often fail.
- Algorithmic Bias Mitigation: Addressing biases in AI to prevent unfair or unethical trading practices.
- Financial Inclusion: Using AI to extend financial services to underbanked areas by assessing creditworthiness through alternative data sources.
- Forex Trading: AI’s application in foreign exchange markets, where geopolitical and economic factors add layers of complexity.
- Insurance and Risk: AI is being used for more accurate pricing of insurance products and real-time risk assessment.
How to Get Started with AI in Your Trading Strategy
- Education and Learning: Start with foundational courses in AI and finance. Platforms like Coursera or edX offer relevant courses.
- Simulation and Back testing: Use platforms like Quantopian or TradingView to test AI strategies in a simulated environment.
- Tools and Platforms: Look into AI trading bots or platforms like TradeStation or Meta Trader which support algorithmic trading.
- Community Involvement: Join forums like Reddit’s r/algo trading or attend webinars to learn from peers and experts.
- Ethical Consideration: Ensure your approach to AI trading includes ethical considerations, particularly around fairness and transparency.
(Also read: Revolutionary AI Tools of 2025: The Top 10 You Need to Know)
Conclusion
AI and Big Data are not just tools but are transforming the very essence of stock trading, making it more accessible, efficient, and potentially more profitable. However, with great power comes great responsibility; ethical use, regulatory compliance, and a balanced approach between human and AI insights are crucial.